Monday, August 24
Monday, August 24 10:00 - 10:10 (America/New_York)
Conference Opening
Join virtual session (via Zoom): https://us02web.zoom.us/j/83805535204
Meeting ID: 838 0553 5204
Password: sent to registered attendees
Monday, August 24 10:10 - 11:10 (America/New_York)
K1: Keynote by Daniel Serfaty, Chairman & CEO at Aptima, Inc.: "Dawn of the Multi-Species Systems: Human Performance Engineering in the Age of AI" 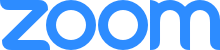
Join virtual session (via Zoom): https://us02web.zoom.us/j/83805535204
Meeting ID: 838 0553 5204
Password: sent to registered attendees
Monday, August 24 11:10 - 11:20 (America/New_York)
Bio Break
Monday, August 24 11:20 - 1:00 (America/New_York)
S1 (Oral Presentation): Situation Assessment and Prediction
Join virtual session (via Zoom): https://us02web.zoom.us/j/83805535204
Meeting ID: 838 0553 5204
Password: sent to registered attendees
- S1.1 Online Intention Recognition Applied to Real Simulated Driving Maneuvers
- Oral PresentationAdvanced Driver Assistance Systems (ADAS) are systems developed to assist the human driver and therefore to make driving safer. Research and development of human driving intention recognition (behavior prediction) plays an important role in the development of ADAS, because the estimated driving intention can be used to supervise the drivers intended actions and to avoid dangerous situations. In this contribution, an approach is developed based on fuzzy-Random Forest (fuzzy-RF) for recognizing human driving intentions in real time. Three different driving maneuvers including left/right lane change (LCL/LCR) and lane keeping (LK) are modeled as classes. Driving simulations are generated for highway scenes using a driving simulator. To improve the recognition performance of the proposed approach, membership functions are applied to quantify input signal data into fuzzy sets for RF training. The design parameters of membership functions can be generated automatically by a fuzzy density clustering method. Using experimental data from real human driving, driver intentions are predicted. The results show accuracy values of off-line training phase and on-line test phase are larger than 98 % and 91 % respectively. The effectiveness of driving intention recognition has been successfully proved in this contribution.pp. 1-6
- S1.2 Situation Alignment for Distributed Operations
- Oral Presentation1In the absence of network and processing limitations, sharing all of the data collected to other nodes in the network will allow all nodes to produce the same understanding of all situations ongoing in the operating environment, i.e., all nodes situation awareness will be in alignment. However, when there are network or processing limitations across the network, not all collected data will be able to be shared to all nodes. This research is concerned with determining the subset of collected data that should be shared across the bandwidth constrained network in order to best align situational understanding across nodes of the network. Four mathematical formulations are derived, making use of Dempster-Shafer calculus, and applied to a illustrative example problem.pp. 7-11
- S1.3 Decision Making with "Gray Swan"events
- Oral Presentation1"Grey Swan" has been defined by Nassime Talib as a rare and highly consequential event that, unlike absolutely unforeseen Black Swan events, can be expected. This paper describes an approach to designing a decision support system, which monitors uncertain and ambiguous dynamic environments characterized by both regular and gray swan events. It is capable of producing probable explanations of the situations, identifying asymmetric threats, and acting to prevent catastrophic consequences or mitigate their impact while operating under resource and time constraints. The proposed system incorporates decision makers' attitudes toward risk and uncertainty while considering regular and gray swan events characterized by uncertain catastrophic outcomes. Use case results are also presentedPresenter bio: Dr. Roman Ilin is a Research Computer Scientist, EO Exploitation Branch, Layered Sensing Exploitation Division, Sensors Directorate, Air Force Research Laboratory, Wright-Patterson Air Force Base, Ohio. Dr. Ilin conducts basic research in the areas of sensor data processing and machine learning. His current research interests are in developing novel algorithms for automatic target extraction and classification from EO and IR imagery and fusion of evidence from multiple information sources. Prior to his current assignment he worked as an NRC Research Associate, at Sensors Directorate, Hanscom Air Force Base, Massachusetts. As a recipient of the National Academy of Sciences Research Associates Program award, he conducted independent research in radar and EO signal processing, developed algorithms for target identification, tracking, classification, and fusion of information from multiple heterogeneous sources.pp. 12-19
- S1.4 Strands of Memory
- Oral Presentation1Software-intensive systems in difficult environments often have to operate without timely human control. That level of autonomy argues for systems that have highly capable self- and situation-awareness processes, to protect the system, perform its tasks, and also to predict its external environment, so that appropriate plans can be made as far in advance as possible, and modified as conditions change. For this level of adaptability, we have long advocated self-modeling systems, which are systems that have models of their behavior, that they interpret to produce that behavior. That means that changing the models changes the behavior. In this paper, we describe two aspects of the memory in such systems, as reflected in two properties about the management of situation awareness: · How to navigate the world and not get surprised (very much). · How to recognize situations when they recur (soon enough to do something useful). Our conclusion is that the system must maintain many many "strands" of memory, that is, partially completed stories about what the environment has done, is doing, and is expected to do, and each one of the strands is (usually implicitly) waiting for some kind of extending, supporting, confirming, or refuting observations or other evidence. The details to be determined are about what those observations can be, what levels of abstraction are required, how they are determined, how the stories are managed, and how they relate to each other. This paper is a first step in that direction.Presenter bio: Dr. Landauer is a mathematician (Ph.D. 1973, Caltech) and co-founder of Topcy House Consulting, working on new theories, methods, practices, and tools for software and system integration. He was at The Aerospace Corporation from 1981 to 2019, and has worked on a wide range of mathematical applications, computational studies, modeling and simulation programs, program and design reviews, tool developments and evaluations, and has most recently worked on new Mathematical methods for data analytics in telemetry analysis, verification and validation of rule-based systems, and how to make sense of incomplete data. He has over sixty years experience in applying mathematical methods to computing problems, with over 190 related publications in mathematics, computer science, computational semiotics, and applied linguistics.pp. 20-27
Monday, August 24 1:00 - 1:01 (America/New_York)
Adjourn for the day
Tuesday, August 25
Tuesday, August 25 8:00 - 9:00 (America/New_York)
K2: Keynote by Prof. Edward Waltz, Naval Postgraduate School, Monterey, CA: "Learning, Reasoning, and Fusion in Human-Machine Teams" 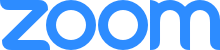
Join virtual session (via Zoom): https://us02web.zoom.us/j/83805535204
Meeting ID: 838 0553 5204
Password: sent to registered attendees
Tuesday, August 25 9:00 - 9:10 (America/New_York)
Bio Break
Tuesday, August 25 9:10 - 10:50 (America/New_York)
S2 (Oral Presentation): Collaborative Decision Making
Join virtual session (via Zoom): https://us02web.zoom.us/j/83805535204
Meeting ID: 838 0553 5204
Password: sent to registered attendees
- S2.1 Prioritizing Policy Objectives in Polarized Groups Using Artificial Swarm Intelligence
- Oral PresentationGroups often struggle to reach decisions, especially when populations are strongly divided by conflicting views. Traditional methods for collective decision-making involve polling individuals and aggregating results. In recent years, a new method called Artificial Swarm Intelligence (ASI) has been developed that enables networked human groups to deliberate in real-time systems, moderated by artificial intelligence algorithms. While traditional voting methods aggregate input provided by isolated participants, Swarm-based methods enable participants to influence each other and converge on solutions together. In this study we compare the output of traditional methods such as Majority vote and Borda count to the Swarm method on a set of divisive policy issues. We find that the rankings generated using ASI and the Borda Count methods are often rated as significantly more satisfactory than those generated by the Majority vote system (p<0.05). This result held for both the population that generated the rankings (the "in-group") and the population that did not (the "out-group"): the in-group ranked the Swarm prioritizations as 9.6% more satisfactory than the Majority prioritizations, while the out-group ranked the Swarm prioritizations as 6.5% more satisfactory than the Majority prioritizations. This effect also held even when the out-group was subject to a demographic sampling bias of 10% (i.e. the out-group was composed of 10% more Labour voters than the in-group). The Swarm method was the only method to be perceived as more satisfactory to the "out-group" than the voting group.pp. 28-36
- S2.2 SAHRTA: A Supervisory-Based Adaptive Human-Robot Teaming Architecture
- Oral PresentationSupervisory-based human-robot teams are deployed in various dynamic and extreme environments (e.g., space exploration). Achieving high task performance in such environments is critical, as a mistake may lead to significant monetary loss. Task performance may be augmented by adapting the supervisory interface's interactions or autonomy levels based on the human supervisor's workload level, as workload is related to task performance. Typical adaptive systems rely solely on the human's overall or cognitive workload state to select what adaptation strategy to implement; however, overall workload encompasses many dimensions (i.e., cognitive, physical, visual, auditory, and speech) called workload components. Selecting an appropriate adaptation strategy based on a complete human workload state (rather than a single workload dimension) may allow for more impactful adaptations that ensure high task performance. A Supervisory-Based Adaptive Human-Robot Teaming Architecture (SAHRTA) that selects an appropriate level of autonomy or system interaction based on a complete real-time multi-dimensional workload estimate and predicted future task performance is introduced. SAHRTA was shown to improve overall task performance in a physically expanded version of the NASA Multi-Attribute Task Battery.pp. 37-44
- S2.3 Comparison of Fuzzy-based Value of Information to Bayesian Inference in a Military Domain
- Oral PresentationDetermining the relative importance of vast amounts of individual pieces of information presents a significant challenge in the military intelligence domain. Using Subject Matter Expert (SME) knowledge to create decision support tools requires aggregation of the various experts' knowledge. The goal of this research endeavor is to investigate a Bayesian inference model for aggregating opinions of military intelligence analysts with respect to the Value of Information (VoI) problem. This paper discusses ongoing VoI research and presents results from an experiment using the Bayesian Thurstonian ranking aggregation model. The Thurstonian rankings are compared to the "ground truth" as generated by the current fuzzy-based VoI prototype system. Results demonstrate the usefulness of the Thurstonian method in aggregating SME opinions and clearly demonstrate the well-know "wisdom of the crowd" effect. Implications related to ongoing VoI research are discussed along with future research plans.pp. 45-52
- S2.4 Training and Verbal Communications in Human-Autonomy Teaming Under Degraded Conditions
- Oral PresentationVerbal communication is important for coordination and performance in many team settings. However, the inclusion of autonomous agents presents challenges to teamwork. This study sought to examine the effects of three different training approaches on team communication behaviors in human-autonomy teams (HAT)s under normal and degraded conditions. Teams were split into three conditions prior to execution of missions in a remotely piloted aircraft system task environment: coordination training, calibration training, and control training. Analysis of text communications indicated that teams that received coordination training pushed information more frequently compared to teams that received control or calibration training, though this effect appeared to diminish over time. Teams in all three conditions pulled information equally, with a reduction in frequency over time. It was also found that teams that received coordination training were more likely to overcome failures attributed to the autonomous agent's cognitive capacities. This research suggests that coordination training in HATs may be effective at influencing team verbal behaviors, however the extent of this effect requires further examination.pp. 53-58
Tuesday, August 25 10:50 - 10:55 (America/New_York)
Bio Break
Tuesday, August 25 10:55 - 11:35 (America/New_York)
PV (Poster Presentation): Poster Preview
Join virtual session (via Zoom): https://us02web.zoom.us/j/83805535204
Meeting ID: 838 0553 5204
Password: sent to registered attendees
Tuesday, August 25 11:35 - 11:45 (America/New_York)
Bio Break
Tuesday, August 25 11:45 - 1:00 (America/New_York)
S3 (Oral Presentation): Machine Learning
Join virtual session (via Zoom): https://us02web.zoom.us/j/83805535204
Meeting ID: 838 0553 5204
Password: sent to registered attendees
- S3.1 Capacitive Swipe Gesture Based Smartphone User Authentication and Identification
- Oral PresentationWeaknesses in smartphone security pose a severe privacy threat to users. Currently, smartphones are secured through methods such as passwords, fingerprint scanners, and facial recognition cameras. To explore new methods and strengthen smartphone security, we developed a capacitive swipe based user authentication and identification technique. Swipe is a gesture that a user performs throughout the usage of a smartphone. Our methodology focuses on using the capacitive touchscreen to capture the user's swipe. While the user swipes, a series of capacitive frames are captured for each swipe. We developed an algorithm to process this series of capacitive frames pertaining to the swipe. While different swipes may contain different numbers of capacitive frames, our algorithm normalizes the frames by constructing the same number of frames for every swipe. After applying the algorithm, we transform the normalized frames into gray scale images. We apply principal component analysis (PCA) to these images to extract principal components, which are then used as features to authenticate/identify the user. We tested random forest (RF) and support vector machine (SVM) algorithms as classifiers. For authentication, the performance of SVM (tested with left swipes) was more promising than RF, yielding a maximum accuracy of 79.88% with an FAR and FRR of 15.84% and 50%, respectively. SVM (tested with right swipes) produced our maximum identification accuracy at 57.81% along with an FAR and FRR of 0.60% and 42.18%, respectively.Presenter bio: Md S. Hossain is an Associate Professor of Computer Science at Southern Connecticut State University, New Haven, CT, USA. He received the B.S. degree in Computer Science and Engineering from the University of Dhaka, Bangladesh, in 2006. He received the M.S. degree in Computer Science and Ph.D. degree in Computational Analysis and Modeling from Louisiana Tech University, Ruston, LA, USA, in 2012 and 2014, respectively. His research interests include multi-biometric verification, behavioral biometrics, user authentication in smartphones, machine learning, and computer vision. He is a member of IEEE.pp. 59-66
- S3.2 An Artificial Intelligence Algorithm to Automate Situation Management for Operators of Unmanned Aerial Vehicles
- Oral PresentationUAV operators must maintain high levels of situation awareness on their area of operation. To achieve this, they use the Command and control (C2) maps, which are shared among forces, and regularly overloaded with data that is irrelevant to their operational mission. UAV operators' require distilled information at the right timing. Yet, the existing filtering mechanisms for C2 maps are layer-based and insufficient. We propose a new approach to automatically and dynamically filter information items on the map based on environmental and mission context. To achieve this, we introduce a three-tiers artificial intelligence (AI) based algorithm (GiCoMAF), where we delineate the use of machine learning (ML) models to support UAV missions. For the GiCoMAF development, tagged data was collected in simulated experimental runs with professional UAS operators. Different types of ML models were evaluated and fitted into the algorithm. The models achieved a relatively high accuracy at modeling human preference and area of interest. The approach presented in this study can be further implemented to support other operators in time-critical spatial-temporal problems.pp. 67-75
- S3.3 Reinforcement Learning Meets Cognitive Situation Management: A Review of Recent Learning Approaches from the Cognitive Situation Management Perspective
- Oral Presentation2With Reinforcement Learning (RL), artificial agents learn reaching their goals "in the wild", i.e., from interacting with their environments. By learning to perform the correct action(s) in the given situation, RL thus adopts an action or decision-centric problem orientation. Conversely, the field of Cognitive Situation Management (CogSiMa), more originating from the control field, focuses on managing the encountered situations, i.e., environment states, such that the desired goal situations are reached or maintained. Whereas both fields of research thus appear complementary in pursuing similar overall goals, RL and CogSiMa have largely evolved independently from each other, leading to terminological gaps, misconceptions and unawareness of potentially related research. The present review attempts to bridge these gaps by providing an integrated framework highlighting the intersections between RL and CogSiMa: We outline how RL in real-world problem domains relates to CogSiMa, aim to bridge the terminological gaps between these distinct communities, and hope to provide the grounding for a cross-fertilization between these distinct research areas. We contribute a review of recent RL developments and discuss their implications and potential for CogSiMa.pp. 76-84
Tuesday, August 25 1:00 - 1:01 (America/New_York)
Adjourn for the day
Wednesday, August 26
Wednesday, August 26 10:00 - 11:00 (America/New_York)
P1 (Poster Presentation): Poster Session
Join virtual session (via Zoom):
General session room: https://us02web.zoom.us/j/83805535204
Meeting ID: 838 0553 5204
Passwords: sent to registered attendees
Individual virtual poster rooms:
P1.1 Autonomy Levels for Small Satellite Clusters: enter poster room (via Zoom): https://us02web.zoom.us/j/ID modified
P1.2 Benefits of Multisensory Cues in a Realistic Target Discrimination Task: enter poster room (via Zoom): https://us02web.zoom.us/j/ID modified
P1.3 Common Alerting Protocol Compliant Emergency Warning and Alert System for Legacy Broadcasting Networks: enter poster room (via Zoom): https://us02web.zoom.us/j/modified ID
P1.4 Human Online Reliability Estimation Applied to Real Driving Maneuvers: enter poster room (via Zoom): https://us02web.zoom.us/j/ID changed
P1.5 Exploring Participants' Views of Cybersecurity Competitions Through the Lens of Social Media: enter poster room (via Zoom): https://us02web.zoom.us/j/ID modified ID
P1.6 From Cyber Incidents to Training Cognitive Situation Management: enter poster room (via Zoom): https://us02web.zoom.us/j/ID modified
P1.8 Understanding the Contextualization of Information in Complex Military Decision Spaces: enter poster room (via Zoom): https://us02web.zoom.us/j/ID modified
P1.9 Degrees of Information Relevance in Situation Assessment: enter poster room (via Zoom): https://us02web.zoom.us/j/ID modified
P1.10 Situational Hazard Recognition and Risk Assessment Within Safety-Driven Behavior Management in the Context of Automated Driving: enter poster room (via Zoom): https://us02web.zoom.us/j/ID modified
P1.11 Feedback Generation Using Fuzzy Cognitive Maps to Reduce Dropout in Situation-Aware e-Learning Systems: enter poster room (via Zoom): https://us02web.zoom.us/j/bbb
P1.12 Autonomic Activity and Surgical Flow Disruptions in Healthcare Providers During Cardiac Surgery: enter poster room (via Zoom): https://us02web.zoom.us/j/ID modified
P1.13 Digital Cognitive Aids to Support Adaptation of Surgical Processes to COVID-19 Protective Policies: enter poster room (via Zoom): https://us02web.zoom.us/j/modified ID
P1.14 A 7-Dimensional Framework for Technical Data in High-Intensity Vital Environments and Its Application to Aircraft Maintenance: enter poster room (via Zoom): https://us02web.zoom.us/j/a
- P1.1 Autonomy Levels for Small Satellite Clusters
- Poster PresentationThe growth in commercial and government use of small satellites and CubeSat constellations is projected to grow significantly over the next decade due to their low cost and flexibility attributes. However, managing clusters of small satellites requires some level of distribution of cognition amongst the elements of the cluster to enable autonomous and robust operations. The goal of introducing cognition is to reduce the latency and increase the accuracy of detections and recognition of objects or events of interest as well as continued proper operation in the event of single or multiple sensor dropouts or mission ground station failures. We propose here an autonomy model that consists of eight levels of autonomy: Remote operation, onboard sensor processing, formation flight, dynamic retasking, onboard sensemaking, dynamic supervised replanning, and dynamic unsupervised replanning. Since space applications have the most stringent constraints on size, weight, and power, it is critical to make decisions that address where the data is stored and how it is processed. We have therefore designed a model that enables specific cognitive functions to be performed on the available data in order to enable the machine decisions that distinguish between the different levels of autonomy.Presenter bio: Bio for Will Williamson Dr. Will Williamson is an Associate Research Professor in the Electrical and Computer Engineering Department of the Naval Postgraduate School (NPS). He holds a PhD in experimental Physics from the University of Toledo. He has worked extensively with the Defense Advanced Projects Agency (DARPA), in a variety of roles over 15 years. He has worked for Center for Naval Analysis, Institute for Defense Analysis, DARPA, and MITRE Corporation on research projects for each of the Armed Services, and several intelligence agencies. He currently supports the NPS Center for Multi-INT Studies in the Washington, DC region. His current research interests are in applying machine learning and artificial intelligence to various defense and intelligence applications including: cyber intrusion detection, characterization of social media influence operations, and multi-INT data fusion.pp. 135-138
- P1.2 Benefits of Multisensory Cues in a Realistic Target Discrimination Task
- Poster PresentationThis study is part of large project aiming at developing brain-computer interfaces capable of enhancing decision-making performance in multisensory environments. In this paper we present results from an experiment exploring the possibility of increasing accuracy of users engaged in the discrimination of difficult realistic targets, in the presence of multisensory cues. More specifically, in our experiment users are asked to discriminate between target individuals rapidly appearing in a indoor environment. Auditory or audio-visual cues may precede the target, warning the users about location of the imminent target. Results show that if the timing of audio-visual cues is right, they can be effective at preparing users to respond to the imminent target, compared to audio-only cues, thereby increasing user performance.pp. 139-143
- P1.3 Common Alerting Protocol Compliant Emergency Warning and Alert System for Legacy Broadcasting Networks
- Poster PresentationIn case of a natural or human intervened disaster and other similar emergency situations, damage to life and property can be minimized if there is a system or mechanism for proper dissemination of information and alerts so that mitigative and preventive measures can be taken well in time by population. Unfortunately existence of such emergency warning alert systems is rare and if they do exist their effectiveness raises concerns. This outreach of emergency messages to the masses can be made more effective if national broadcasting agencies are involved. All India Radio (AIR) is India's national broadcaster. When it comes to reaching out to masses via radio network, All India Radio has the largest outreach in the world. As All India Radio has a broad coverage of population, a CAP compliant system incorporated with broadcasting organization has a greater impact on population. This feature can be implemented with digital radios but changing the complete infrastructure posses huge cost investment. This paper proposes a low cost Common Alerting Protocol (CAP) compliant system for All India Radio with no or minimal impact on AIR legacy infrastructure. The system being proposed possesses compliance with Common Alerting Protocol which is an Extensible Markup Language (XML) based data format for exchanging public warning and emergency messages between alerting technologies. The proposed system extracts information embedded in a CAP message and makes it available to the AIR broadcasting system which is then disseminated using its transmitters. The system is also designed to display the message on public display screens. E-mails are also sent to concerned authorities. The proposed system follows a multichannel approach for information dissemination.pp. 144-148
- P1.4 Human Online Reliability Estimation Applied to Real Driving Maneuvers
- Poster PresentationTraffic safety is mainly affected by human factors and related human reliability. During the driving process, effects of human factors, such as fatigue and vigilance, on driving safety have been widely discussed. Human reliability is less considered, especially in situated driving context. Human reliability is a commonly used concept in probability assessment context. Many approaches have been developed in human reliability analysis (HRA). Cognitive reliability and error analysis method (CREAM) provides a human cognitive model called contextual control mode (COCOM) and a method to describe and evaluate the most significant factors in context as common performance conditions (CPCs). Based on CREAM, reliability of human operators can be evaluated. However, due to the application limits of CPCs in CRERAM, it is advised to generate a new list of CPCs for the application domain, if the CREAM approach is applied to other domains. In this contribution, a new approach defining a situated and dynamical human reliability measure is established. The approach is based on the well-known CREAM approach, which is modified with respect to the use in dynamical context. The new list of CPCs is generated to illustrate the features of situated driving context. Furthermore the reliability estimation is understood as a dynamic task with changing conditions leading to a dynamical change of reliability properties. Driving data collected by driving simulator are processed, the effects of experimental sequences and scenarios on reliability of human drivers are analyzed. Reliability of human drivers in situated driving context can be estimated by the newly introduced human performance reliability score (HPRS). The results indicate the applicability of the proposed approach. The new approach first time realizes the evaluation of human online reliability in situated driving context.pp. 149-154
- P1.5 Exploring Participants' Views of Cybersecurity Competitions Through the Lens of Social Media
- Poster PresentationCybersecurity competitions have been recognized as a great approach to enhancing cybersecurity education. As the industry fights to solve the cybersecurity workforce shortage, various cybersecurity competitions have been developed. Although several studies have investigated the characteristics of the competition participants based on self-reported data, there remains a great need to gain a better understanding of the participants' views from an objective standpoint. In this study, we investigate the participants' comments, interests, and engagement based on the evidence collected from multiple social media platforms, including Reddit, Twitter, and Google Trends. Given the collected data, we conducted a content analysis to identify popular topics discussed in Reddit communities through the use of coding. Besides, we analyzed Twitter and Google Trends data to investigate the trends of people's interests and sentiment of multiple aspects. Our analysis results enable us to discuss several practical implications of the findings.pp. 155-162
- P1.6 From Cyber Incidents to Training Cognitive Situation Management
- Poster PresentationHeterogeneous interacting socio-technical systems, distinct by their own cultures, structures, methods, and machines, are transforming the process of making informed decisions and assessment of situations in the context of cybersecurity into a serious challenge. Stakeholders in each system are forced to take actions at any given time based on the situation. These actions not only affect their own, but also the whole system's properties. Therefore, maintaining cybersecurity will become more difficult. In this work in progress, we attempt to apply a cognitive learning model in agent-based computational economics to create a comprehensive environment for cybersecurity situation modeling, reasoning and learning. Taken together, this enables us to holistically assess the situations and systems' reflexivity, and support human and/or computational understanding of the situations. The aim of this study is to design and develop a hands-on cybersecurity platform to train cognitive situation management in cyber ranges.pp. 163-166
- P1.7 Biologically-Inspired Personalities for Control Systems and Robots Using Nonlinear Optimization and Feedback Theory
- Poster PresentationThis paper presents a quadrant system and mathematical model for describing the clinical personality disorders, human personality self-images, and human personality behaviours. A proposed biological mechanism that would result in this personality structure means the system can be understood as plausible from first principles. Then similarities of self-images to Q and R in an LQR optimization, and the similarities of behavioural techniques to the gains in a PID feedback control, allow a construction of a computer model. Validity is tested with nonlinear optimization to predict the probability distribution of human behavioural techniques, as described by the Myers-Briggs Temperament Indicator, for different self-images. The results closely match that previously reported from human data. The model directly results in the ability to design control systems with personalities and shows how one would approach programming personalities on robots and chat-bots.Presenter bio: Chris Macnab received her B.Eng. from the Royal Military College of Canada in Engineering Physics in 1993. She completed a Ph.D. at the University of Toronto Institute for Aerospace Studies in 1999. Her topic was stable neural-network control of space manipulators with joint flexibility. She is currently an Associate Professor in the Department of Electrical and Computer Engineering at the University of Calgary. Her research is focused on putting personalities on robots and chat-bots.pp. 167-174
- P1.8 Understanding the Contextualization of Information in Complex Military Decision Spaces
- Poster PresentationOne of the central challenges associated with developing situational understanding originates at the information level. The simple fact is while all information may be created equal, the value of that information is not. Confounding this challenge is the fact that the true value of information is dependent not only on its source and latency, but just as importantly on the context in which it is being exercised. Towards this end, this paper presents a multi-faceted experiment meant to discern how Soldiers weigh the value of information against seven contextual variables under varying military scenarios. Initial results reveal that contextual variables play a significant role in how information is valued and more importantly provide a foundation for developing tailorable information strategies, increasing situational awareness, and reducing information- and cognitive-overload.pp. 175-179
- P1.9 Degrees of Information Relevance in Situation Assessment
- Poster PresentationInformation overload is a big challenge that the decision makers need to overcome while assessing and sharing information about the situations they are dealing with. In our previous papers, we developed an information relevance reasoning method to support the human decision makers to identify the relevant information that needs to be processed. However, the amount of the relevant information identified by this method still may grow fast when large volumes of information are continuously added to the knowledge base. The collected information selected by this method would include redundant facts, which are relevant to a situation, but not necessary for understanding the situation. In order to identify only the information that is necessary for characterizing a specific situation, we need to refine the relevant information and remove the redundant part. In this paper, we first extend the conceptual framework for the identification of information relevance developed in our previous papers by considering degrees of relevance. Based on the definition of weak relevance and strong relevance, we develop relevant information simplification algorithms to remove the redundant facts from the relevant facts. We evaluate our method in a medical cyber security scenario. The results show that our relevant information simplification method reduces the size of the information for characterizing a specific situation and the amount of time that is needed to infer answers to queries related to the situation. We also verify that by removing the redundant relevant information we can get the same answers to the queries as if using the whole knowledge base.pp. 180-187
- P1.10 Situational Hazard Recognition and Risk Assessment Within Safety-Driven Behavior Management in the Context of Automated Driving
- Poster PresentationThis paper addresses the problem of hazard recognition and risk assessment in open and non-predictive environments to support decision making and action selection. Decision making and action selection incorporate decreasing situational risks and maintain safety as operational constraints. Commonly, neither existing application-related safety standards nor the situation modeling or knowledge representation is considered in that context. This contribution introduces a novel approach denoted as a Safety Driven Behavior Management focusing on situation modeling and the problem of knowledge representation in its sub-functions in the context of situational risks. It combines the safety standards-oriented hazards analysis and the risk assessment approach with the machine learning-based situation recognition. An example illustrating the approach is presented in this paper.pp. 188-194
- P1.11 Feedback Generation Using Fuzzy Cognitive Maps to Reduce Dropout in Situation-Aware e-Learning Systems
- Poster PresentationE-learning is becoming increasingly popular among learners of any age, thanks to its many advantages related to the possibility to learn anywhere and anytime and the low costs. Despite this success and diffusion, the student dropout rate of e-learning systems is very high. The main reason is the lack of motivation and engagement of the students with the online course. An adequate design of the e-learning system and its capability to adapt to the characteristics of the learners can reduce the dropout rate. In this work, we propose an approach, based on Fuzzy Cognitive Map, to identify the situation of the learner (mainly in terms of motivation and engagement) and to provide the learners with a set of feedback aiming at improving the retention of the learners in a situation-aware adaptive learning system. A prototypical system is proposed to preliminary verify the feasibility and utility of the approach.Presenter bio: Giuseppe D'Aniello received the Master's degree in Computer Engineering in 2013 and the Ph.D. degree in Information Engineering in 2018, both from the University of Salerno, Italy. He is a postdoc research fellow (RTD-A) at the University of Salerno. He was an Executive Researcher and Project Manager at the CO.RI.SA. (Research Consortium on Agent System). He is a member of the IEEE SMC TC on Cognitive Situation Management. His current research interests include situation awareness, computational intelligence, and granular computing. He has co-authored several scientific papers on these topics, published in international journals and international conference proceedings.pp. 195-199
- P1.12 Autonomic Activity and Surgical Flow Disruptions in Healthcare Providers During Cardiac Surgery
- Poster PresentationCardiac surgery represents a complex sociotechnical environment relying on a combination of technical and non-technical team-based expertise. Surgical flow disruptions (SFDs) may be influenced by a variety of sources, including social, environmental, and emotional factors affecting healthcare providers (HCPs). Many of these factors can be readily observed, except for emotional factors (i.e. distress), which represents an underappreciated yet critical source of SFDs. The objective of this study was to demonstrate the feasibility of detecting autonomic nervous system (ANS) activity as a proxy for emotional distress associated with a known near-miss event using heart rate variability (HRV) analysis. Audio/video and HRV were captured intraoperatively and time-synchronized. Following a critical medication administration error by the anesthesiologist in-training, data sources were consulted to identify events precipitating this near-miss event. Using pyphysio, an open-source physiological signal processing package, we analyzed the attending anesthesiologists' HRV, specifically the low-frequency high-frequency (LF/HF) ratio (normal value < 2) as a primary indicator of sympathetic nervous system (SNS) activity and thereby emotional distress, and the root mean square of the successive differences (RMSSD) as a primary indicator of parasympathetic nervous system (PNS) activity. A heightened SNS response in the attending anesthesiologists' physiological arousal, observed as an LF/HF ratio value of 3.39, was detected prior to the near-miss event. Coinciding with this was a sharp decrease in PNS activity. The attending anesthesiologist confirmed a state of high distress induced by task-irrelevant environmental factors. Qualitative analysis of audio/video recordings revealed that the ANS activation detected was temporally associated with an argument over operating room management occurring at the same time as the LF/HF ratio elevation. This study confirms that it is possible to recognize detrimental psychophysiological influences in cardiac surgery procedures via advanced HRV analysis. To our knowledge, ours is the first such case demonstrating ANS activity coinciding with strong self-reported emotion during live surgery using HRV. Despite extensive experience in the cardiac OR, transient but intense emotional changes may have the potential to disrupt attention processes in even the most experienced HCP. A primary implication of this work is the possibility to detect real-time ANS activity, which could enable personalized interventions to proactively mitigate downstream adverse events. Additional studies on our large database of surgical cases are underway and new studies are actively being planned to confirm this preliminary observation.pp. 200-204
- P1.13 Digital Cognitive Aids to Support Adaptation of Surgical Processes to COVID-19 Protective Policies
- Poster PresentationSurgical processes are rapidly being adapted to address the COVID-19 pandemic, with changes in procedures and responsibilities being made to protect both patients and medical teams. These process changes put new cognitive demands on the medical team and increase the likelihood of miscommunication, lapses in judgment, and medical errors. We describe two process model driven cognitive aids, referred to as the Narrative View and the Smart Checklist View, generated automatically from models of the processes, that can support the introduction and use of these new processes. The immediate perceived utility of these cognitive aids is as instruments to support medical simulation and adaptations to changing operating room guidelines.pp. 205-210
- P1.14 A 7-Dimensional Framework for Technical Data in High-Intensity Vital Environments and Its Application to Aircraft Maintenance
- Poster PresentationAircraft maintenance crews interact with a large and complex data ecosystem. Data is generated in the form of procedural manuals, inspection notes and reports, task orders, and more, but it is largely stove-piped, resulting in inadequate access, duplication of efforts, a lack of data pedigree and traceability, and hidden anomalies. This paper describes a conceptual metadata framework designed to enable efficient and effective reasoning over that information. In turn, it is expected to optimize data availability and presentation to maintainers, when they need it, in the format most appropriate, and in the right context. Additionally, we anticipate this metadata framework to permit proficient leveraging of existing data to make predictions about what maintenance procedures should be performed and when.pp. 211-215
Wednesday, August 26 11:00 - 11:10 (America/New_York)
Bio Break
Wednesday, August 26 11:10 - 12:50 (America/New_York)
S4 (Oral Presentation): Situation Monitoring
Join virtual session (via Zoom): https://us02web.zoom.us/j/83805535204
Meeting ID: 838 0553 5204
Password: sent to registered attendees
- S4.1 Cognitive Cloud to Edge Systems for Remote Real Time Monitoring: CO2 Sensing at Mount Nyiragongo Volcano, Employing the Internet Backpack
- Oral PresentationCloud technologies and edge (Internet of Things) devices and services have been widely utilized to improve safety and community resiliency. However, in regions with low Internet penetration or even in well-connected communities temporarily 'off-grid,' accessing and coordinating these tools can be a challenge. The Conflict Zone volcano monitoring application explored in this paper may be considered an extreme case. The authors argue that the successful approach assessed in this paper may apply not only to uncommon circumstance. This paper focuses on the use of the Internet Backpack technology as a core part of a cloud to edge communication and information system for monitoring sensors for early detection of CO2 levels in the city of Goma, in the Democratic Republic of the Congo. Goma is located dangerously close to Mt. Nyiragongo, the third most dangerous volcano in the world. Deadly CO2 emissions in the surrounding areas and Lake Kivu require continuous monitoring by the Goma Volcano Observatory (OVG). The authors describe testing, implementation and challenges in the mountainous terrain encountered and overcome with the Internet Backpack, in cooperation with the OVG.Presenter bio: Lee W.McKnight is an Associate Professor in the iSchool (The School of Information Studies), Syracuse University, Faculty Advisor to the Worldwide Innovation Technology and Entrepreneurship Club (WiTec), and an Affiliate of the Institute for National Security and Counterterrorism (INSTC). Lee lectures annually at MIT since 1998. Lee was Principal Investigator of the 2011 Technology Project of Year TACNY Award-winning National Science Foundation Partnerships for Innovation Wireless Grids Innovation Testbed (WiGiT) project 2009-2014. Lee is inventor of edgeware, a new class of software for creating secure ad hoc overlay cloud to edge applications, services, and Things. Lee's research focuses on blockchain and cloud management of cyber-physically secure dynamic edge services, virtual markets and wireless grids, the global information economy, national and international technology policy, and Internet governance.pp. 85-89
- S4.2 Distributed Multi-Objective Cooperative Coevolution Algorithm for Big-Data-Enabled Vessel Schedule Recovery Problem
- Oral PresentationDuring a maritime voyage, delays due to disruptive events could result in financial and reputation losses. The vessel schedule recovery problem (VSRP) aims at adjusting vessel speeds to mitigate the negative impact of such delays. The granulated speed-based vessel schedule recovery problem (G-S-VSRP) is a big-data-enabled VSRP. It is a multiobjective optimization problem defined by dividing the trajectory between ports into regions (encoded by geohashed system) and mining the speed profiles in these regions from Automatic Identification System (AIS) data. The G-S-VSRP minimizes delay and financial loss of a vessel voyage; it also maximizes the speed compliance with the historical navigational patterns. Using geohash-based speed mining on AIS data in the G-S-VSRP gives rise to a large-scale optimization problem, where the number of speed variables in geohashed regions grows to the order of thousands. Due to the complexity of such a problem, traditional multiobjective evolutionary algorithms (MOEAs) would stop improving or showing steady behavior. We improve the MOEA's performance using a cooperative coevolution algorithm based on a divide-and-conquer approach to deal with large-scale optimization problems. We introduce a novel Distributed Multiobjective Cooperative Coevolutionary Algorithm (DMOCCA) to improve the performance of MOEAs.pp. 90-97
- S4.3 Hard and Soft Data Fusion for Maritime Traffic Monitoring Using the Integrated Ornstein-Uhlenbeck Process
- Oral PresentationWhen commonly used Automated Identification System (AIS) data to monitor vessel traffic is unavailable, one can resort to other types of data such as remote sensing and open source data to improve detection and tracking in order to achieve or increase Maritime Domain Awareness (MDA). The paper proposes a method for fusion of selected open source (soft), Twitter included, and remote sensing (RADARSAT-2) and AIS-S/T (hard) data in application to maritime traffic monitoring in both coastal and open waters. The method assumes modeling vessels motion by the Integrated Ornstein-Uhlenbeck process. The fusion performance evaluation using real data is presented showing improved tracking and MDA especially in the absence of AIS data. The impact of inclusion of soft, in particular the Twitter data, into the fusion module is also discussed.Presenter bio: Dr. Melita Hadzagic is a Chief Scientist at OODA Technologies. Previously, she worked as a Staff Research Scientist at NATO CMRE, Italy, as a Lecturer at University of Montreal, Dept. of Mathematics and Statistics and a Postdoctoral Researcher at the Centre de recherches mathématiques , Université de Montréal, Canada. She has obtained her Ph.D. and M.Eng. degrees, both in the field of information fusion, from the Dept. of Electrical and Computer Engineering, at McGill University, Montreal, and B.Sc. from the Faculty of Electrical Engineering, University of Belgrade, Serbia. Her research interests include data fusion, predictive modeling, situation management and understanding, control theory, digital signal processing and blockchain. Dr. Hadzagic has over 15 years of experience in R&D of technologies enabled decision support most notably designing and developing solutions which support tactical and strategic maritime situation understanding.pp. 98-105
- S4.4 Predictive Situation Display for Emergency Medical Services: Situational Awareness in Multi-Casualty Incidents
- Oral PresentationThe management of a multi-casualty incident (MCI) requires effective situational awareness. This paper describes the design and test of a situation display for the dispatch center of emergency medical services during the MCI. The display consists of areas showing the amount and locations of vehicles en-route to the incident area, statistics about vehicles and casualties on site, and state of hospitals and evacuating vehicles. The display also includes predictive elements. The study shows that the presence of the predictive elements facilitated more correct responses to situational awareness probes, particularly those requiring projection. In addition, the findings show that the predictive elements facilitated more correct responses to situation management questions.pp. 106-112
Wednesday, August 26 12:50 - 1:00 (America/New_York)
Bio Break
Wednesday, August 26 1:00 - 1:30 (America/New_York)
Invited Talk by Prof. Ben Shneiderman, University of Maryland, MD: "Human-Centered Artificial Intelligence: A Second Copernican Revolution" 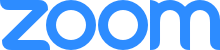
Join virtual session (via Zoom): https://us02web.zoom.us/j/83805535204
Meeting ID: 838 0553 5204
Password: sent to registered attendees
Wednesday, August 26 1:30 - 1:31 (America/New_York)
Adjourn for the day
Thursday, August 27
Thursday, August 27 8:00 - 9:00 (America/New_York)
K3: Keynote by Prof. Barry Smith, University at Buffalo, NY: "Command and Control" 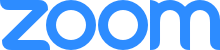
Join virtual session (via Zoom): https://us02web.zoom.us/j/83805535204
Meeting ID: 838 0553 5204
Password: sent to registered attendees
Thursday, August 27 9:00 - 9:10 (America/New_York)
Bio Break
Thursday, August 27 9:10 - 10:25 (America/New_York)
S5 (Oral Presentation): Applications
Join virtual session (via Zoom): https://us02web.zoom.us/j/83805535204
Meeting ID: 838 0553 5204
Password: sent to registered attendees
- Situation Awareness and Information Fusion in Sales and Customer Engagement: A Paradigm Shift
- Oral PresentationWith today's savvy and empowered customers, sales requires more judgment and becomes more cognitively intense than ever before. We argue that Situation Awareness (SA) is at the center of effective sales and customer engagement in this new era, and Information Fusion (IF) is the key for developing the next generation of decision support systems for digital and AI transformation, leveraging the ubiquitous virtual presence of sales and customer engagement which provides substantially richer capacity to access information. We propose a vision and path for the paradigm shift from Customer Relationship Management (CRM) to the new paradigm of SA and IF. We argue this new paradigm solves major problems of CRM paradigm: it reduces the burden of manual data entry and enables more reliable, comprehensive and up-to-date data and knowledge, enhances individual and team SA and alleviates information silos with increased knowledge transferability, and enables more powerful ecosystem of applications by providing common shared layer of computable knowledge assets.pp. 113-121
- Heterogeneous UAV Multi-role Swarming Behaviors for Search and Rescue
- Oral PresentationA swarm of autonomous Unmanned Aerial Vehicles (UAVs) is implemented in simulation to cooperatively gather situational awareness data during the first few hours after a major natural disaster. The swarm is controlled by prioritized sets of reactive behaviors which integrate collision avoidance, battery recharge, formation control, altitude maintenance, methods to optimize the coverage area of of simulated camera mounted on the UAVs. The behaviors are implemented in simulation on swarms of sizes from 10 to 50 UAVs. The UAVs are each assigned one of 3 different roles: social searcher, antisocial searcher, or relay. The simulation uses actual location data, including post-disaster satellite imagery, real locations of damaged and inundated buildings, and realistic victim locations based on personal interviews and accounts. 100 different role distributions are compared over 1000 simulation runs to determine the optimal distribution of UAVs over the target area. The results show that the swarm is successful in locating over 90\% of survivors in less than 35 minutes using the most effective role distributions. Results also show that the addition of any number of relay UAVs decreases performance of the swarm when compared to a distribution that does not include any relays.Presenter bio: Mr. Benjamin Abruzzo is a civilian research engineer for the United States Army and is pursuing a Ph. D. from Stevens Institute of Technology in the field of cooperative robotics. Current topics of interest include heterogeneous teams composed of either wholly autonomous agents or mixed human-robot teams. Additionally, he collaborates with the Robotics Research Center at West Point as a researcher for computer vision and machine learning. Previously Mr. Abruzzo has designed both mechanisms and algorithms for guided munitions while employed at Picatinny Arsenal, NJ and developed perception software for the Robonaut, NASA’s robotic astronaut.pp. 122-128
- Establishing and Maintaining Situation Awareness for Shop-Floor Assistance in Manufacturing
- Oral Presentation4We report on design and implementation of a situation awareness module for a site-wide assistance system in manufacturing plants. We design individual assistance units that are able to support workers interactively, on the shop floor and we enable these units to keep track of the shop-floor situation at the level of workers' cells. Each work cell is thus logically monitored by an assistance unit that is aware of workers, manufacturing tasks and sub-tasks, as well as having an inventory of components and equipment used by the workers, to accomplish their tasks. Input from multiple sensors is translated into streams of observations expressed at a higher conceptual level, so that they can be interpreted w.r.t. a predefined, discrete knowledge model.pp. 129-134
Thursday, August 27 10:25 - 10:35 (America/New_York)
Bio Break
Thursday, August 27 10:35 - 12:25 (America/New_York)
AP: CogSIMA 10th Anniversary Panel 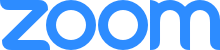
Join virtual session (via Zoom): https://us02web.zoom.us/j/83805535204
Meeting ID: 838 0553 5204
Password: sent to registered attendees
The CogSIMA 10th Anniversary Panel looks on historic roots of the conference, sees what the current successes and pitfalls are, and in what directions the conference should go in the future. The panel gathers widely recognized experts in the field cognitive situation management, who are asked to give their opinion on the above-defined issues from multiple scientific viewpoints, including cognitive science, human factors, psychology, computer science and systems engineering.
Further details: CogSIMA 10th Anniversary Panel
Thursday, August 27 12:25 - 12:45 (America/New_York)
Conference Closing
Join virtual session (via Zoom): https://us02web.zoom.us/j/83805535204
Meeting ID: 838 0553 5204
Password: sent to registered attendees
Thursday, August 27 12:45 - 12:46 (America/New_York)
Adjourn
Friday, August 28
Friday, August 28 10:00 - 2:00 (America/New_York)
W1: Workshop "Interoperability for Situation Management and Decision Making"
Join virtual session (via Zoom): https://us02web.zoom.us/j/83805535204
Meeting ID: 838 0553 5204
Password: sent to registered attendees